2022 Amazon Fellows
The Science Hub advisory group selected 13 Amazon Fellows for 2022 out of 44 nominations. The fellows are PhD students in the departments of computer science, electrical and computer engineering, mechanical and aerospace engineering, and bioengineering in the UCLA Samueli School of Engineering.
The 2022 Amazon Fellows presented their research during the Lightning Talks event held on Feb 23, 2023. To view the Lightning Talks video or their individual presentation decks, click here.

Sanae Amani Geshnigani
Advisor: Lin Yang
Electrical and Computer Engineering
I develop bandit and reinforcement learning (RL) algorithms for safety-critical decision-making, online advertising, multi-agent and lifelong systems. More specifically, I have worked on bandits in both single-agent and distributed multi-agent settings, safe and lifelong RL with function approximation, and multinomial logistic regression bandits with applications in online advertising.
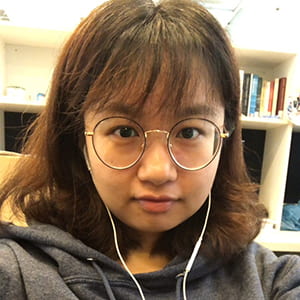
Kewei Cheng
Advisor: Yizhou Sun
Computer Science
My research interest mainly focuses on graph learning and machine learning in general with a particular interest in neural-symbolic knowledge graph reasoning.
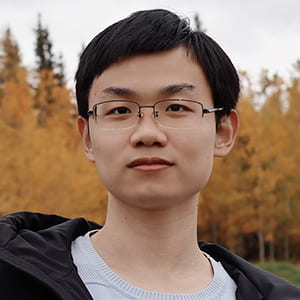
Zi-Yi Dou
Advisor: Nanyun (Violet) Peng
Computer Science
I am interested in connecting language to perception and action. In particular, I have worked on learning vision-and-language representations through pre-training and how embodied agents can utilize the learned representations for downstream tasks.
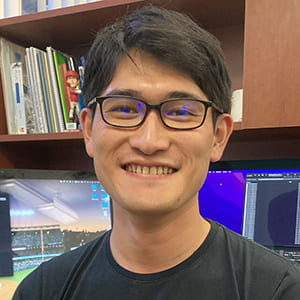
Kai Fukami
Advisor: Prof. Kunihiko Taira
Mechanical and Aerospace Engineering
My research interest focuses on developing physics-inspired data-driven techniques for turbulent flow analyses, leveraging computational fluid dynamics, super/unsupervised machine learning, and complex network theory.
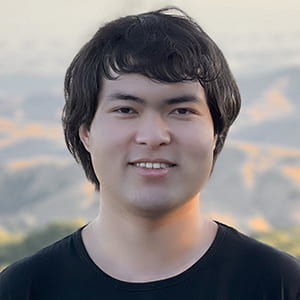
Luzhe Huang
Advisor: Aydogan Ozcan
Electrical and Computer Engineering
I’m devoted to building advanced computational and microscopy imaging technologies using disciplinary knowledge in AI and optics. I also aim to investigate more robust, generalizable and interpretable DL models.
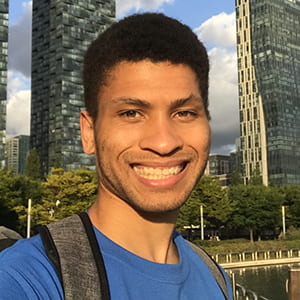
Alexander Johnson
Advisor: Abeer Alwan
Electrical and Computer Engineering
Inclusive speech technology for low-resource dialects and populations including automatic speech recognition for African American English and children’s speech.
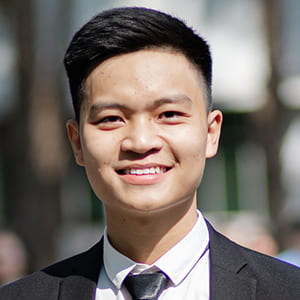
Tung Nguyen
Advisor: Aditya Grover
Computer Science
My research interest lies in the intersection of sequence modeling, uncertainty quantification, and decision making. I am also interested in grounding my foundational research in applications to sustainability development, especially tackling climate change.
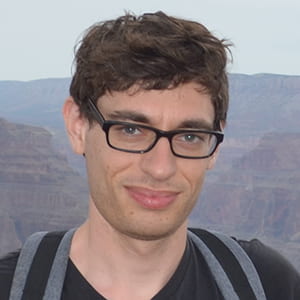
Alexander Schperberg
Advisor: Dennis Hong
Mechanical and Aerospace Engineering
I formulate software architectures which combines traditional control schemes with vision and machine-learning towards making robots safer and easier to use. Recently, I have been working on auto-tuning methods to self-calibrate low-level controllers and high-level planning algorithms for stable and energy efficient motion planning of legged, aerial, and wheeled robots.
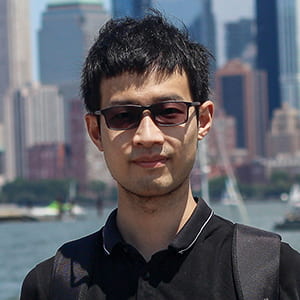
Zhouxing Shi
Advisor: Cho-Jui Hsieh
Computer Science
I am interested in trustworthy machine learning, including evaluating and verifying the robustness of machine learning models, and training robust models.
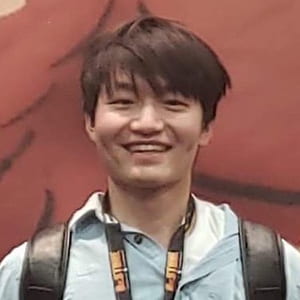
Zhaoqiang Wang
Advisor: Liang Gao
Bioengineering
My research interest lies in optical systems and computational imaging. Currently, I am focusing on high-speed 3D microscopy with machine learning to address the transient volumetric biological process (e.g. blood blow, neural signals)
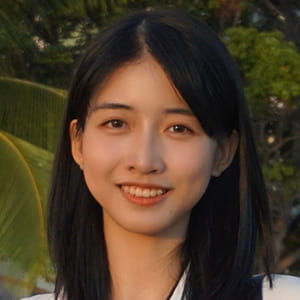
Yu Yang
Advisor: Baharan Mirzasoleiman
Computer Science
My research primarily focuses on understanding and improving the efficiency and accountability of machine learning. In particular, I am developing scalable methods to improve the in- and out-of-distribution generalization performance of the models, as well as their robustness against spurious correlations and malicious examples.
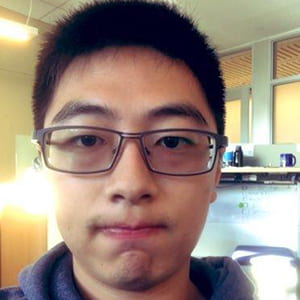
Da Yin
Advisor: Kai-Wei Chang
Computer Science
My research interest is knowledge acquisition and building knowledge-enhanced AI models. Currently, I am focusing on evaluating geographical inclusivity of AI models and enhancing it with knowledge.
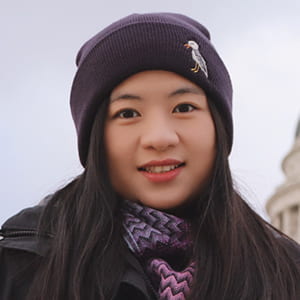
Zhe Zeng
Advisor: Guy Van den Broeck
Computer Science
I am interested in enabling machine learning systems to efficiently and reliably learn and infer from noisy, structured, and mixed discrete-continuous data. I approach this goal using probabilistic modeling, statistical relational learning, approximate inference, and formal methods.