2023 Amazon Fellows
Congratulations to the 11 graduate students from UCLA Samueli School of Engineering who have been selected by the Science Hub Advisory Group as the 2023 Amazon Fellows. The selection process was not an easy one with a pool of 24 highly accomplished nominees. Although an award could not be made to all, we commend all of the nominees for their outstanding accomplishments thus far.
The 2023 Amazon Fellows presented their research during the Lightning Talks event held on Jan 25, 2024. To view the Lightning Talks video or their individual presentation decks, click here.

Ulzee An
ADVISOR: Sriram Sankararaman
Computer Science
My research interests are in developing deep learning based methods that can accurately and efficiently learn from all available information in large clinical databases in the presence of varying degrees of availability and modalities of data.

Evan Becker
ADVISOR: Alyson Fletcher
Computer Science
The broad goal of my research is to expand our understanding of non-convex optimization procedures in modern ML methods, particularly in generative models. A key focus is in the high-dimensional regime, where mathematical concentration results can provide precise and insightful analyses.
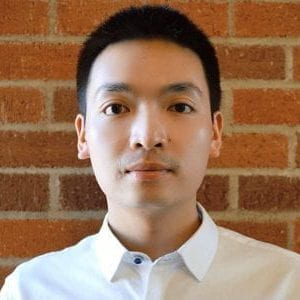
Guorui Chen
ADVISOR: Jun Chen
Bioengineering
My research focuses on leveraging bioelectronics and artificial intelligence to address unsolved challenges in healthcare. Recently, I have been using machine learning to interpret biosensor data for identifying cardiovascular disease phenotypes, aiming to advance personalized medicine and improve patient outcomes.

Jiafan He
ADVISOR: Quanquan Gu
Computer Science
I am highly interested in the fields of optimization and machine learning, with a particular focus on reinforcement learning. I am driven to explore the theoretical foundations of reinforcement learning and develop data-efficient algorithms that can address real-world challenges effectively.
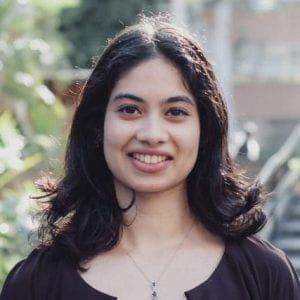
Roshni Iyer
ADVISORS: Yizhou Sun and Wei Wang
Computer Science
My research interest lies in the intersection of machine learning and data mining, where I develop models for heterogeneous information networks. To this end, I am developing efficient graph embedding methods for improved representation of knowledge graphs, social networks, and natural language.

Xingyu “Bruce” Liu
ADVISOR: Xiang “Anthony” Chen
Electrical and Computer Engineering
My research interests lie at the intersection of Human-Computer Interaction (HCI) and AI. Specifically, I design, implement and evaluate systems that support and augment human’s communication abilities. My research presents Human-AI systems that enable people to communicate with richer information, higher efficiency, and better accessibility.
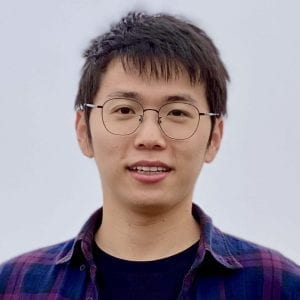
Pan Lu
ADVISORS: Song-Chun Zhu and Kai-Wei Chang
Computer Science
My research interests lie in natural language processing and machine reasoning, aiming to develop machines capable of human-like reasoning and collaboration. Currently, I am focusing on developing large language models in mathematical and scientific domains, as well as multi-modal settings.

Varuni Sarwal
ADVISOR: Eleazar Eskin
Computer Science
My research interests lie in Artificial Intelligence (AI) for healthcare. I am particularly focused on 1) Developing deep learning-based scalable models for time series Electronic Health Record (EHR) datasets and 2) Improving model interpretability of time-based explainers.

Yufei Tian
ADVISOR: Nanyun (Violet) Peng
Computer Science
My research interest lies in natural language processing, especially creative (and controllable) text generation including lyrics, poems, and humor generation, commonsense and logical reasoning, and evaluation metrics for NLG. I also investigate how to inject human behaviors into language models.

Yihan Wang
ADVISOR: Cho-Jui Hsieh
Computer Science
My research interest is machine learning, especially evaluating and improving machine learning models across different data distributions such as adversarial data and unseen tasks.
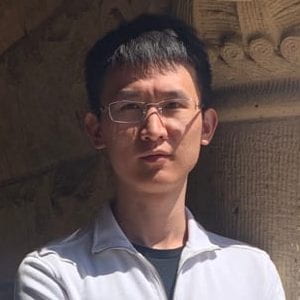
Shichang Zhang
ADVISOR: Yizhou Sun
Computer Science
My research interest lies in developing efficient and explainable machine learning models for graph data. Specifically, I have focused on accelerating and explaining graph neural networks with applications in large-scale online classification, efficient and explainable recommendation systems, and drug discovery.