2022 Research Project Funding Recipients
The Science Hub advisory group selected 6 research projects for funding in 2022. The investigators are professors in the Samueli School of Engineering and the David Geffen School of Medicine.
Investigator

Wei Wang
Leonard Kleinrock Chair Professor in Computer Science
Director of Scalable Analytics Institute
Department of Computer Science
Department of Computational Medicine
UCLA
Research project
Knowledge Graph Representation Learning and Applications in Biomedicine
We aim to develop a framework for integrated modeling through representation learning, which will be further extended to do representation learning on heterogeneous graphs and to support dynamic graphs.
Learn more...
Knowledge graphs have been widely adopted as a universal representation that bridges the gap between data and knowledge. However, most current knowledge graph models have one or both of the following limitations. (a) Most models focus on instance-level knowledge. There lacks integrated modeling of the instance-level and concept-level knowledge graphs. (b) Data/knowledge are assumed to be static (or with rare updates), which does not hold in many applications where data and knowledge may accumulate rapidly. However, temporality is crucial in many applications. We hereby propose to develop a framework for integrated modeling through representation learning. Our approach is innovative and transformative, benefiting various downstream tasks. Our research and innovations can be thus organized into the following research goals.
(1) Knowledge Graph Representation Learning. We introduce a novel method to derive hierarchical knowledge graph representations. These embedding vectors provide a seamless integration of the instance-level knowledge with the concept hierarchies (e.g., ontology) of the instance-level entities.
(2) Graph Representation Learning with Heterogeneous and Dynamic Properties. Knowledge graphs often involve extremely complicated heterogeneous and dynamic properties in the real world. We propose a generative model to capture the system dynamics of complicated knowledge graphs over time.
(3) Universal Framework for Real-world Applications. While preliminary studies with promising results validate each proposed component, our representation learning framework and comprehensive graph embeddings are actually universal for myriad useful applications with machine learning optimization.
Investigator

Baharan Mirzasoleiman
Assistant professor, Computer Science
Research project
Coresets for Efficient and Robust Machine Learning
Our main objective is to develop practical and theoretically rigorous methods that enable efficient and robust learning from massive datasets. We will address this problem by carefully selecting subsets of training data that warrant superior generalization and robustness properties.
Learn more...
The great success of modern machine learning systems is contingent on exceptionally large computational resources that enable training complex models on abundant data. However, this incurs substantial financial and environmental costs and is susceptible to low-quality labeled examples. While datasets are steadily growing, their information volume is much smaller than their data volume due to quality issues and redundancies in big data. Hence, the entire data volume is not always required to train
accurate models. To improve scalability and robustness of machine learning, it becomes crucial to develop methods that can make efficient use of big data, by accurately and robustly learning from the information volume. However, the high-dimensional and non-convex nature of modern machine learning models, in particular deep networks, makes developing such methods
very challenging.
Our main objective is to tackle the above challenge by developing practical and theoretically rigorous methods that enable efficient and robust deep learning from massive datasets. To achieve this, we will leverage properties of the loss landscape associated with individual examples at different points during the training. This allows us to theoretically quantify the value of different subsets of data points for training and optimization, by utilizing higher-order interactions between examples. Based on the above idea, we will extract the information volume, by identifying examples that provably contribute the most to learning and safely excluding those that are redundant or mislabeled. Training on the extracted information volume allows us to efficiently learn models with better generalization and robustness properties.
Investigators
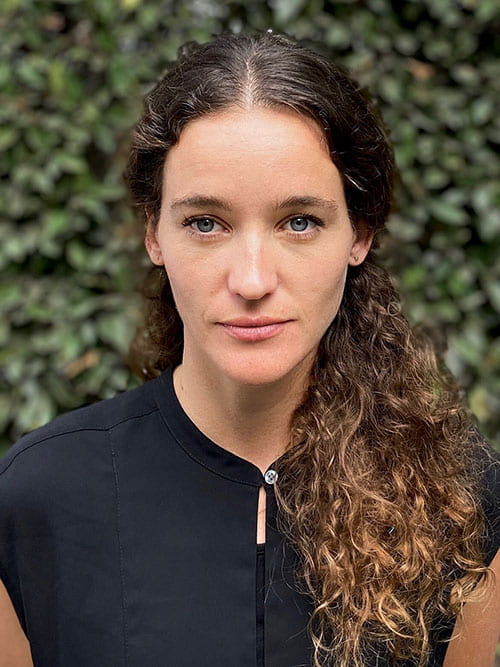
Loes Olde Loohuis, Ph.D.
Assistant Professor of Psychiatry & Biobehavioral Sciences and Human Genetics, David Geffen School of Medicine at UCLA
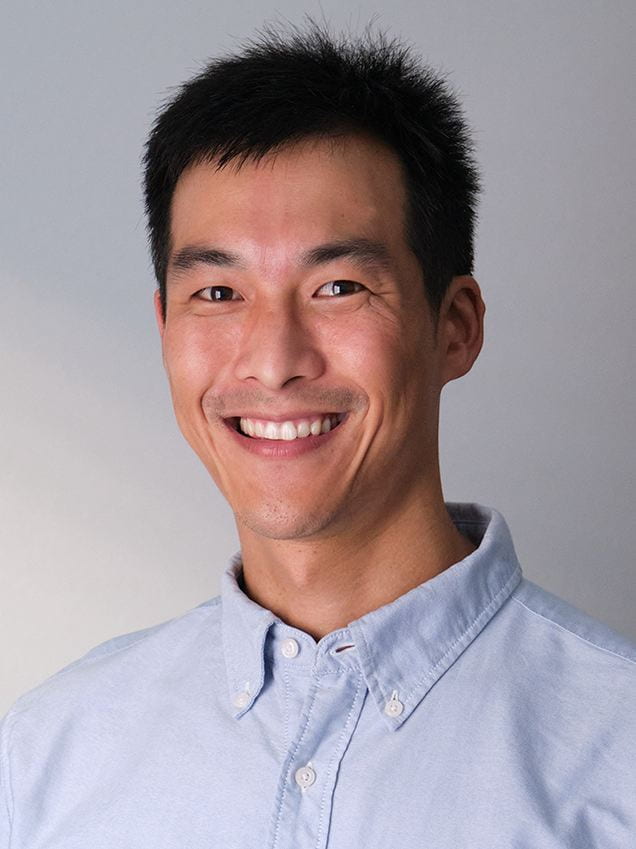
Jeffrey Chiang, Ph.D.
Assistant Adjunct Professor
Research project
Prediction of Perinatal Depression Using EHR-derived Phenotypes and Genetic Risk Scores
We will leverage the UCLA Health research infrastructure to develop a predictive model for depressive illness occurring during pregnancy or following childbirth, using clinical and genetic predictors.
Learn more...
Perinatal depression (PND), defined as depressive illness occurring during pregnancy or following childbirth, affects between 10-20% of women. It is one of the greatest causes of mortality and morbidity in mothers, including a high risk of suicide, and has detrimental consequences for the child. There is thus an urgent need to identify women at high risk for PND.
PND has been hypothesized to represent a more genetically homogeneous disorder than non-PND depression, occurring in women of reproductive age with onset coupled with pregnancy and childbirth, a time in which the body undergoes tremendous change. Its estimated heritability lies between 40-55%, which is substantially higher than that of (non-PND) depression. Despite these unique factors, PND has traditionally been overwhelmingly understudied compared to other psychiatric disorders.
We will leverage the UCLA Health research infrastructure to develop a predictive model for PND using clinical and genetic predictors. Specifically, we aim to (i) identify at-risk mothers early on during pregnancy and predict time-to-onset; (ii) evaluate whether genetic risk scores generated from existing genome-wide association studies of depression and related mental illnesses can predict PND and/or improve the clinical predictor in patients at clinical high risk; and (iii) compare genetic risk for PND to non-PND depression.
Investigators
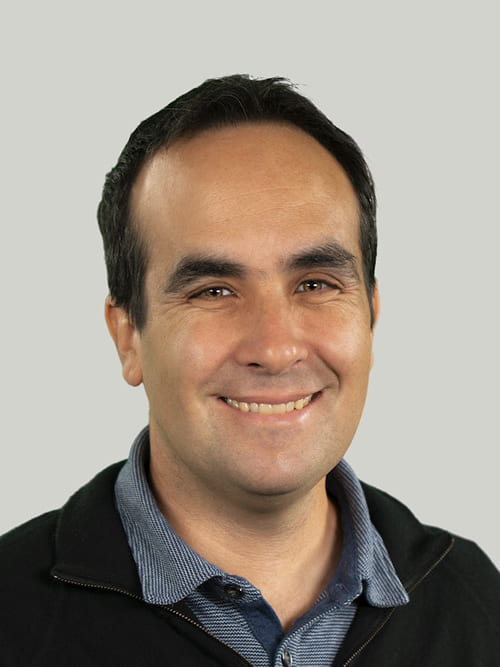
Eleazar Eskin
Professor and Chair, Computational Medicine
Professor, Computer Science
Professor, Human Genetics
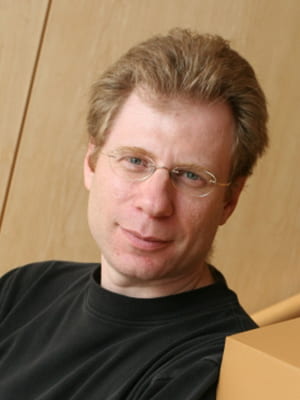
Leonid Kruglyak
Distinguished Professor, Department of Human Genetics
Distinguished Professor, Department of Biological Chemistry
Investigator, Howard Hughes Medical Institute
Founding Member, UCLA Computational Biosciences Institute
The Diller-von Furstenberg Endowed Chair in Human Genetics
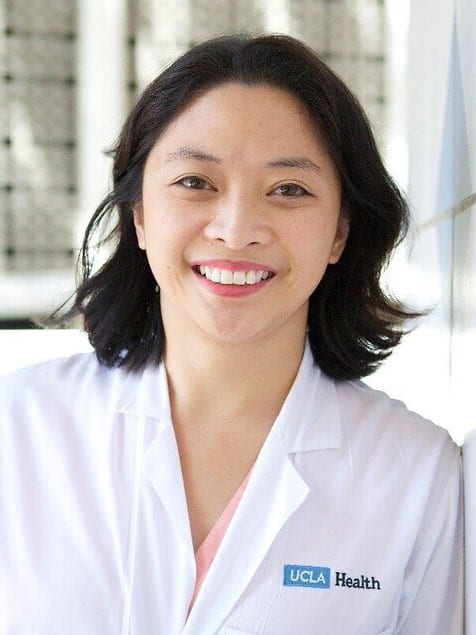
Valerie Arboleda
Assistant Professor, Computational Medicine
Assistant Professor, Human Genetics
Assistant Professor, Pathology
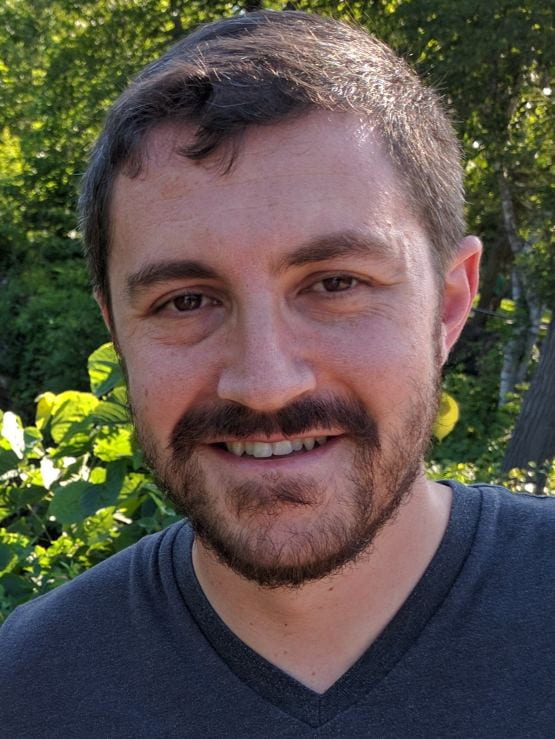
Joshua Bloom
Assistant Adjunct Professor, Computational Medicine

Chongyuan Luo
Assistant Professor, Human Genetics
Research project
Scalable Sequencing Approaches for Detection of Novel Pathogens and Evolving Viral Variants
Swab-Seq is a scalable, inexpensive and accurate COVID-19 Diagnostic testing technology based on next generation sequencing. The goal of this project is to extend Swab-Seq technology to have the capability to detect all known and unknown respiratory technologies at scale.
Learn more...
At UCLA in collaboration with Octant we have developed the Swab-Seq COVID-19 Diagnostic technology based on next generation sequencing technology. Swab-Seq is scalable, inexpensive and accurate. The method uses the power of next generation sequencing technology to analyze thousands of samples simultaneously. The technology labels each person’s sample with a unique piece of DNA that acts as a molecular barcode. A polymerase chain reaction (PCR) amplifies nucleic acid in each sample, including any virus it might contain, and DNA sequencing is used to detect those samples with virus, assigning the virus to the individuals it came from on the basis of the molecular barcodes. Swab-Seq has processed over 1,000,000 tests. The goal of this project is to extend Swab-Seq technology to have the
capability of detecting all respiratory viruses at scale. Common respiratory viruses in children below the age of five have a combined global mortality that exceeds 2.5 million deaths each year. As the COVID-19 pandemic has shown, the emergence of a new pathogen can wreak havoc on our society in a very short period of time, especially in the absence of diagnostic capabilities at scale. Early detection and rapid development of diagnostic capabilities are critical to preparedness for the next pandemic.
Investigators

Sriram Sankararaman
Associate Professor of Computer Science, Human Genetics, and Computational Medicine

Tzung K. Hsiai, MD, PhD
Professor of Medicine and Bioengineering
UCLA Cardiovascular Engineering & Light-Sheet Imaging Laboratory
Maud Cady Guthman Endowed Chair in Cardiology
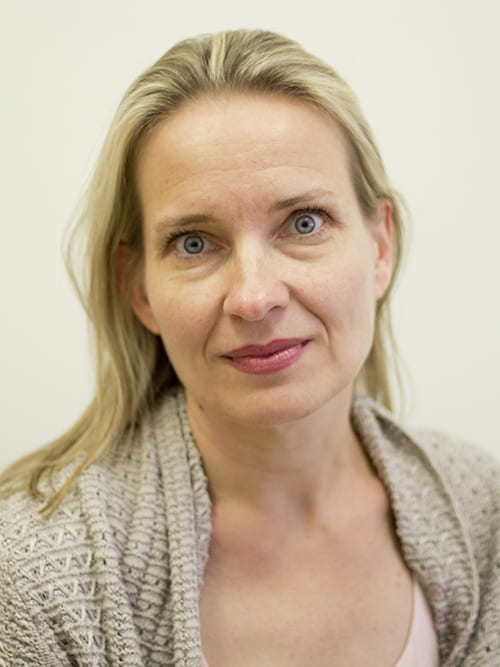
Paivi Pajukanta, MD, PhD
Professor of Human Genetics
Diller-von Furstenberg Family Endowed Chair in Precision Clinical Genomics
Vice Chair, Department of Human Genetics
Director of Cardiometabolic Genomics, Institute for Precision Health
Director of Genetics and Genomics PhD Program
David Geffen School of Medicine at UCLA
Research project
Deep Learning for Biological Discovery with Application to Cardiometabolic Disease
We propose to develop deep learning-based approaches impute missing data and identify disease subtype in large-scale biobank data and to evaluate the utility of these approaches in the context of cardiometabolic disease.
Learn more...
The past decade has seen the growth of large-scale biomedical datasets that collect deep phenotypic and genomic data across individuals. By capturing a wide range of data associated with an individual’s demographic information, laboratory tests, images, medications, disease codes, and genomics, these biobanks can revolutionize biological discovery by providing large sample sizes and enabling the discovery of disease subtypes.
However, biobank data have important limitations that preclude their potential from being fully realized. We propose principled statistical approaches to two key problems in the analysis of biomedical data: the ubiquity of missing data and the identification of disease subtypes. First, we will develop deep learning-based imputation approaches that can express complex, non-linear relationships between the phenotypes while capable of handling structured missingness in incomplete datasets with millions of entries and heterogeneous feature types. Second, we propose to develop feature attribution techniques to interpret the predictions of our deep learning-based imputation techniques and to use these interpretations as the starting point for defining disease subtypes.
While broadly applicable, we will evaluate the validity and utility of the proposed techniques in the context of type 2 diabetes and heart failure: conditions that have a substantial burden on public health and are associated with hard-to-measure risk factors.
Investigators
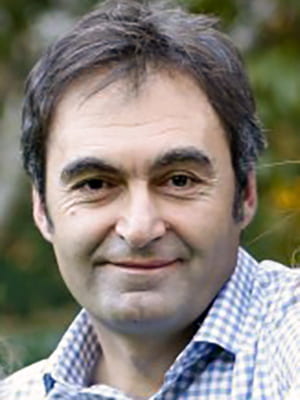
Ertugrul Taciroglu
Professor & Chair
Civil and Environmental Engineering, UCLA
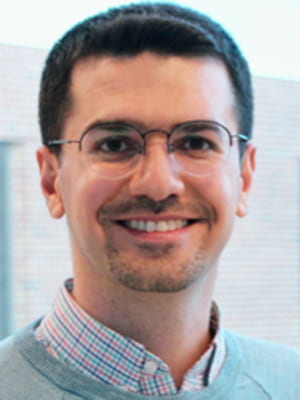
Mohamad Alipour
Research Assistant Professor,
Civil and Environmental Engineering, University of Illinois at Urbana-Champaign
Research project
Fighting Wildfires with AI: Enabling High-Fidelity Wildfire Simulation using Probabilistic Geospatial Deep Learning
This project seeks to advance wildfire management strategies by leveraging AI. We will employ probabilistic geospatial deep learning techniques to produce large-scale real-time wildfire fuel maps that enable and improve disaster mitigation, fire spread simulation, and response measures.
Learn more...
The effects of wildfires on human life and communities as well as the environment, ecosystems, and wildlife habitat are receiving increasing attention due to the unprecedented increases in their frequency and severity. By developing a better understanding of wildfire fuel biomass, our project contributes to improved wildfire modeling and risk assessment, which is important in mitigation, management, and wildfire response. This project seeks to leverage the potential of Artificial Intelligence (AI) to help address this critically urgent problem. To that end, we develop a probabilistic geospatial deep learning framework for quasi-real-time wildfire fuel estimation and mapping. We collect and leverage multimodal remote sensing and biophysical data, and real-world field surveys of biomass to develop models capable of characterizing forest surface and canopy vegetation and combustible biomass that will be inputs to uncertainty-aware fire spread simulations. With the existing shortage of real-time fuel biomass maps across the US, this project will present a unique contribution to the efforts to restore quality of life, environmental justice, and socio-ecological balance within the affected communities.